HPCDataset
We present a large-scale HP counting corpus HPCDataset. To the best of our knowledge, it is the first such corpus. HPCDataset was built based on the data acquired at the Affiliated Zhejiang Hospital, Zhejiang University School of Medicine, China. HPCDataset comes from three data types: 1) Real data; 2) Synthetic data(HP-positive) and 3) Synthetic data(HP-negative).
The source code of this paper is available at PAC-HP.
We collected H&E WSI of 49 gastric biopsies(38 H. pylori-positive and 11 H. pylori-negative biopsies) from 57 patients and each WSI was subdivided into non-overlapping image patches of size 1024 X 1024 pixels. We invited pathologists to extract and annotate the H. pylori from the provided images. After that, a total of 859 H. pylori-positive patches was annotated.
Figure 1. The exemplars of original images from the proposed HPCDataset.
In order to increase the dataset size, we used the proposed image augmentation to construct a synthetic H. Pylori counting dataset. The detailed statistics are reported in Table1. We predict a series of plausible locations according to the experiment of pathology experts, which H. pylori are usually locate at the mucosa and epithelium of gastric tissues. Then the generated H. pylori are placed on the original H. pylori-positive images.
Figure 2. The exemplars of synthetic(HP-positive) images from the proposed HPCDataset.
The generated H. pylori are randomly placed on the light background of the H. pylori-negative images.
Figure 3. The exemplars of synthetic(HP-negative) images from the proposed HPCDataset.
Table 1 shows the basic information of HPCDataset.
Training Data | Number of Images | Average Resolution | Count Statistics | |||
---|---|---|---|---|---|---|
Min | Age | Max | ||||
Real data | 659 | 1024 × 1024 | 1 | 37 | 532 | |
Synthetic(HP-positive) | 1,517 | 1024 × 1024 | 4 | 78 | 632 | |
Synthetic(HP-negative) | 3,400 | 1024 × 1024 | 5 | 44 | 295 | |
Real + Synthetic | 2,176 | 1024 × 1024 | 1 | 65 | 632 |
Table 1. Statistics of the real data and synthetic data.
Precise Augmentation and Counting of Helicobacter Pylori in Histology Image
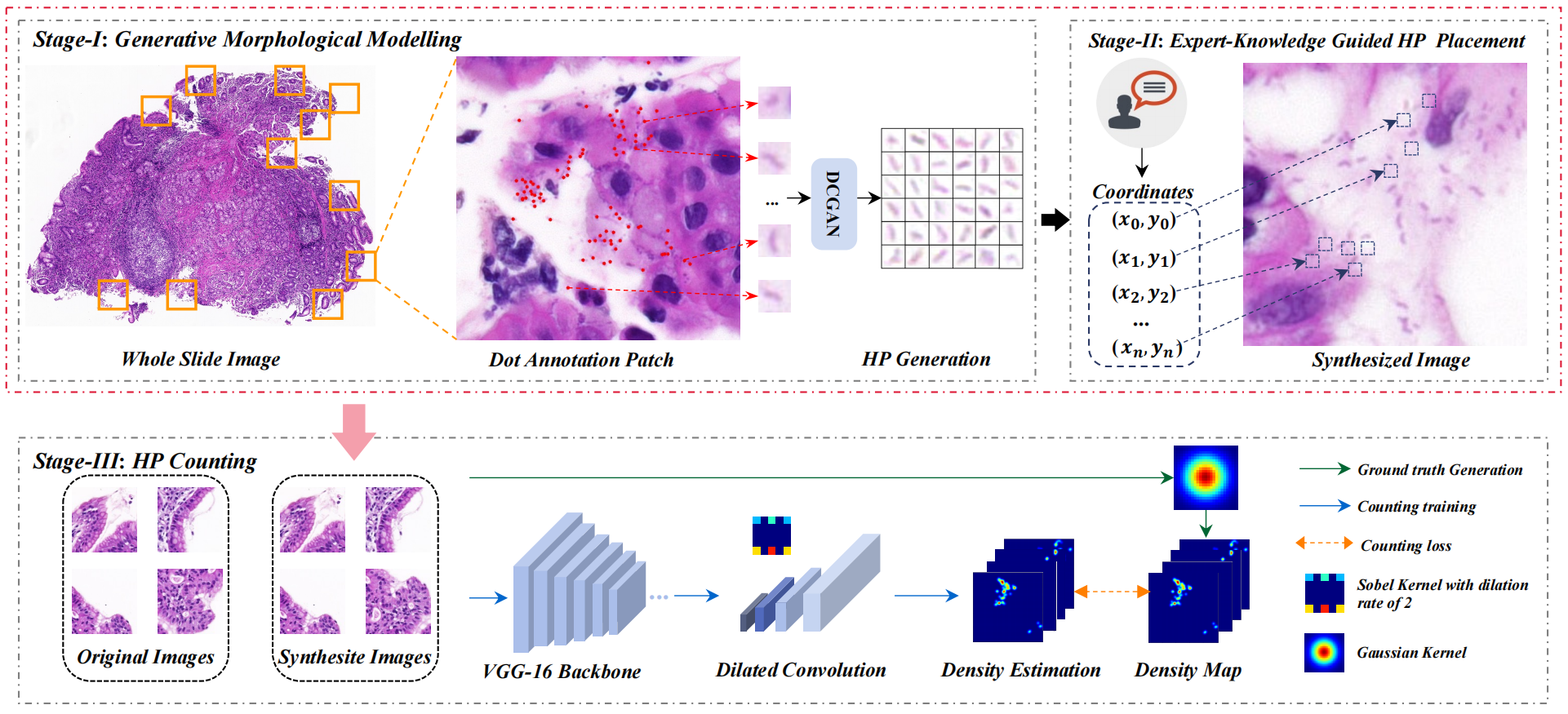
We study the precise counting of Helicobacter Pylori (HP), which is important for diagnosis of gastric cancer. The crowd counting technique is adapted for a precise quantitative analysis. The challenge of training an HP counting model lies in scarcity of labels. We use a DCGAN for the generative modelling of HP morphology and perform high-fidelity data augmentation. The comparative results show our method outperforms the object detection and semantic segmentation baselines. The proposed framework is potential useful in quantitative analysis of other bacteria in histology images.
Bibtex
Please cite the following paper if you use our work.
@inproceedings{neuripsmed2022, title={Precise Augmentation and Counting of Helicobacter Pylori in Histology Image}, author={Cui Y, Chen Y, Shuai Z, et. al.}, booktitle={Proceedings of IEEE Conference on Neural Information Processing Systems (NeurIPS)}, pages={x-x}, year={2022} }